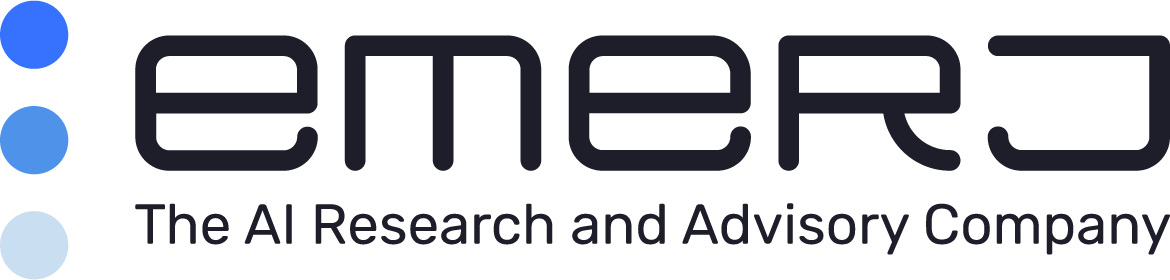
Inference: Where AI Training Ends & Business Begins
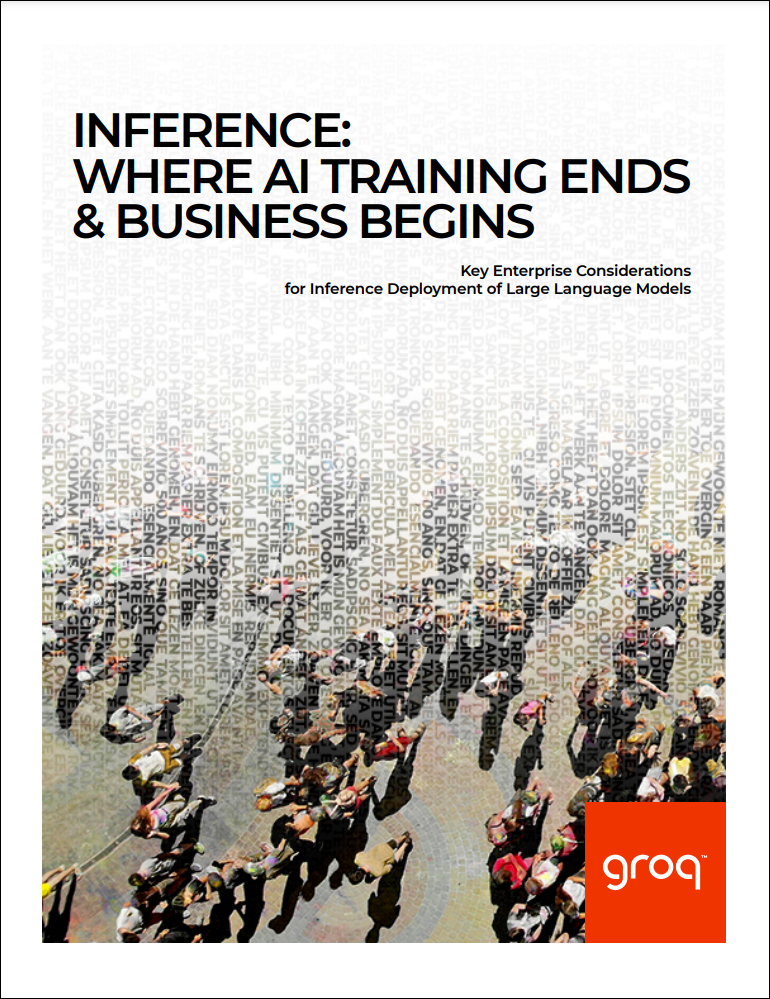
Historically, most artificial intelligence (AI) systems investments have focused on training. We are now at an inflection point where business leaders must move their trained AI models from production to inference.
Groq is a tech company specializing in simplifying compute challenges to accelerate workloads in artificial intelligence, machine learning, and high-performance computing. A new white paper from Groq explains how inference uses input data to solve real-world challenges, enabling businesses to compete in a market abundant with data that demands real-time insights at an accelerated time-to-production.
While training may be the necessary first investment when building an AI strategy, inference turns that data into profit by operationalizing production-ready workloads and models to help with real-world and real-time decision-making.
In this report, you’ll gain insight on:
- Pace
- Predictability
- Performance
- Accuracy
How hardware constrains the rate of LLM innovation and why new model architectures are constantly emerging, both to push the limits of what AI can achieve and to simplify and lower the costs of scaling.
How critical performance metrics such as throughput, latency, accuracy and power consumption can only be measured with determinism – or a system’s ability to deliver predictable and repeatable performance – to guarantee 100% accuracy.
The importance of considering the rate of tokens output per second – not just the rate of tokens input and processed per second – when evaluating inference processors for deploying autoregressive LLMs.
A look at novel, emerging techniques that enable continuous learning as training improves and search-ahead techniques become less effective.
Created by: